Introduction: Generative AI
Word’s out- Generative AI is the single biggest thing happening to tech in a long long time. The market is estimated to be worth around $13 billion, and every day is a day filled with innovations and launches in the tech sector.
Generative artificial intelligence (AI) refers to the branch of AI that focuses on creating new and original content based on existing data or patterns. Unlike traditional AI models that are designed to perform specific tasks or provide predetermined outputs, generative AI models have the ability to generate novel and creative outputs.
Generative AI has attained popularity due to its potential applications in various domains, and its ability to produce content that is indistinguishable from human-created content. This has opened up new possibilities and opportunities in creative fields, allowing artists, musicians, and writers to explore new ideas and push the boundaries of their respective industries.
It is being used in fields such as art, music, literature, and even scientific research. Generative AI models can create realistic images, compose original music pieces, generate new text, and much more.
However, it is important to note that generative AI still faces certain limitations and challenges. One of the key challenges is maintaining control over the generated outputs. As generative AI models become more proficient at generating realistic content, there is a risk of producing misleading or harmful information. Ensuring ethical use and responsible deployment of generative AI is vital to mitigate these risks.
If you want to know more, read here.
Prompting in Generative AI
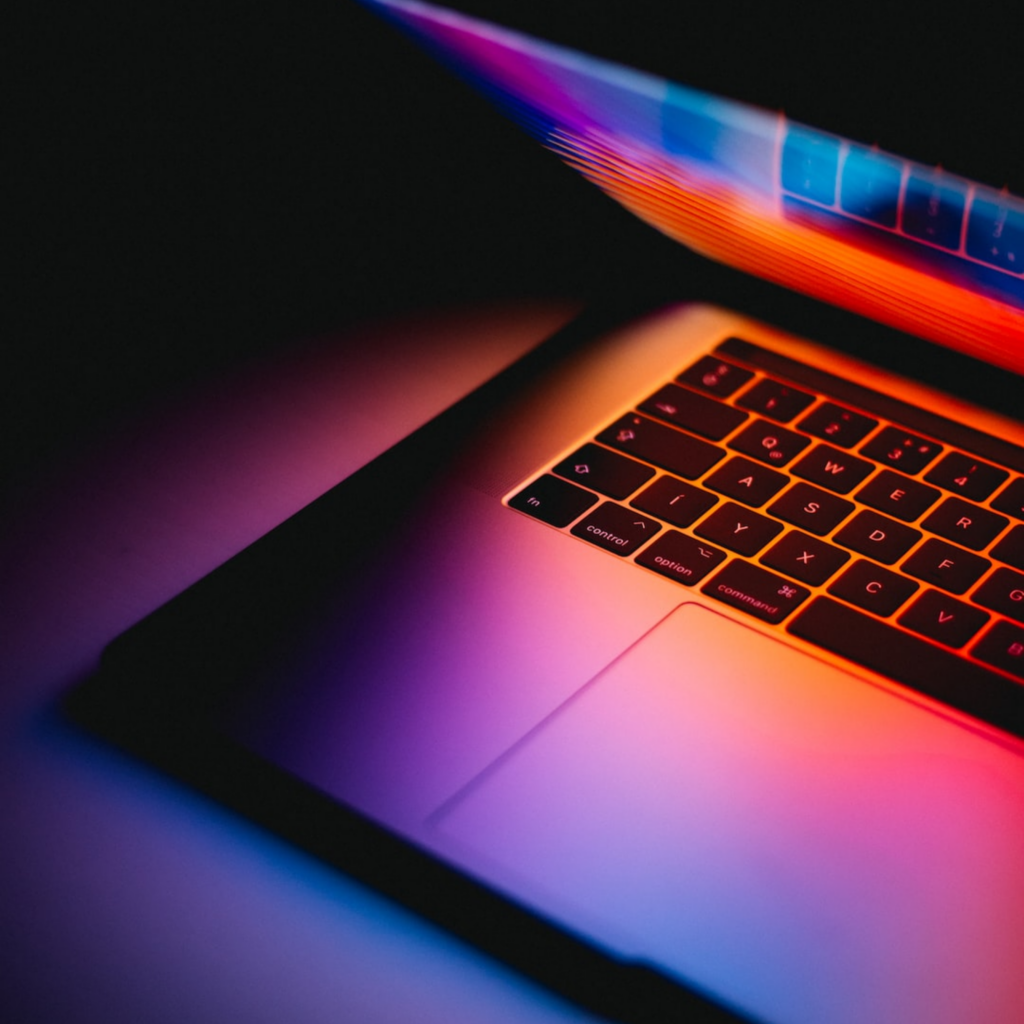
When we talk of generative AI, we have to talk about prompting. Prompting refers to the input or instruction provided to the AI model, which serves as a guide for generating specific outputs. Prompts act as the catalyst for instructing the model’s behaviour and shaping the outcome of its creative processes.
Prompts can take various forms depending on the specific task or application. They can be in the form of a question, a sentence, or even a specific set of keywords. For example, in the context of generating text, a prompt could be as simple as providing the first few words of a paragraph, or it could be a detailed description of the desired content.
One of the key challenges in generative AI is the ability to generate coherent and contextually appropriate outputs. Prompting plays a crucial role in addressing this challenge by providing a framework or direction for the AI model to follow. By carefully crafting prompts, developers can guide the model’s creativity and ensure that the generated content aligns with the desired objectives.
That being said, prompting has certain limitations in the current Generative AI scenario.
- Depending solely on prompts may lead to over-reliance on specific patterns or preconceived notions, resulting in limited creativity and innovation. It is crucial to strike the right balance between providing guidance through prompts and allowing the model to explore new possibilities and generate truly original content.
- Another aspect to consider in the role of prompting is the potential biases introduced through the prompts themselves. The choice of words, phrases, or concepts in the prompts can inadvertently reinforce existing biases or stereotypes present in the training data. Developers must be mindful of these biases and actively work towards creating prompts that promote inclusivity, fairness, and unbiased content generation.
As generative AI continues to advance, the role of prompting may evolve and become more sophisticated. Researchers and developers are exploring new techniques and approaches to enhance the effectiveness of prompting, such as fine-tuning models based on user feedback or incorporating reinforcement learning to improve the responsiveness to prompts.
But this discussion begs an important question: Is prompt engineering the future of AI?
Prompt Engineering: Future of Generative AI?
As generative AI continues to evolve, the role of prompting is likely to remain crucial, but its implementation may undergo significant changes:
- Semantic and Contextual Understanding: Future advancements might enable AI models to better understand the context and nuances of prompts, leading to more accurate and context-aware outputs.
- Few-Shot and Zero-Shot Learning: There is ongoing research in developing AI models that can produce high-quality content with minimal or no explicit prompts, relying on only a few examples to guide the output.
- Human-AI Collaboration: The future might see more collaborative approaches, where humans and AI work together to refine prompts, making the generative process more interactive and creative.
- Prompt Engineering Tools: As the demand for generative AI grows, we can expect the emergence of specialized tools and platforms for prompt engineering, empowering developers and users to achieve more precise outputs.
- Ethical Prompting Guidelines: As AI continues to impact society, there will be an increased focus on developing ethical prompting guidelines to ensure AI-generated content remains within acceptable bounds.
Problem Formulation: A More Holistic Approach Towards Generative AI
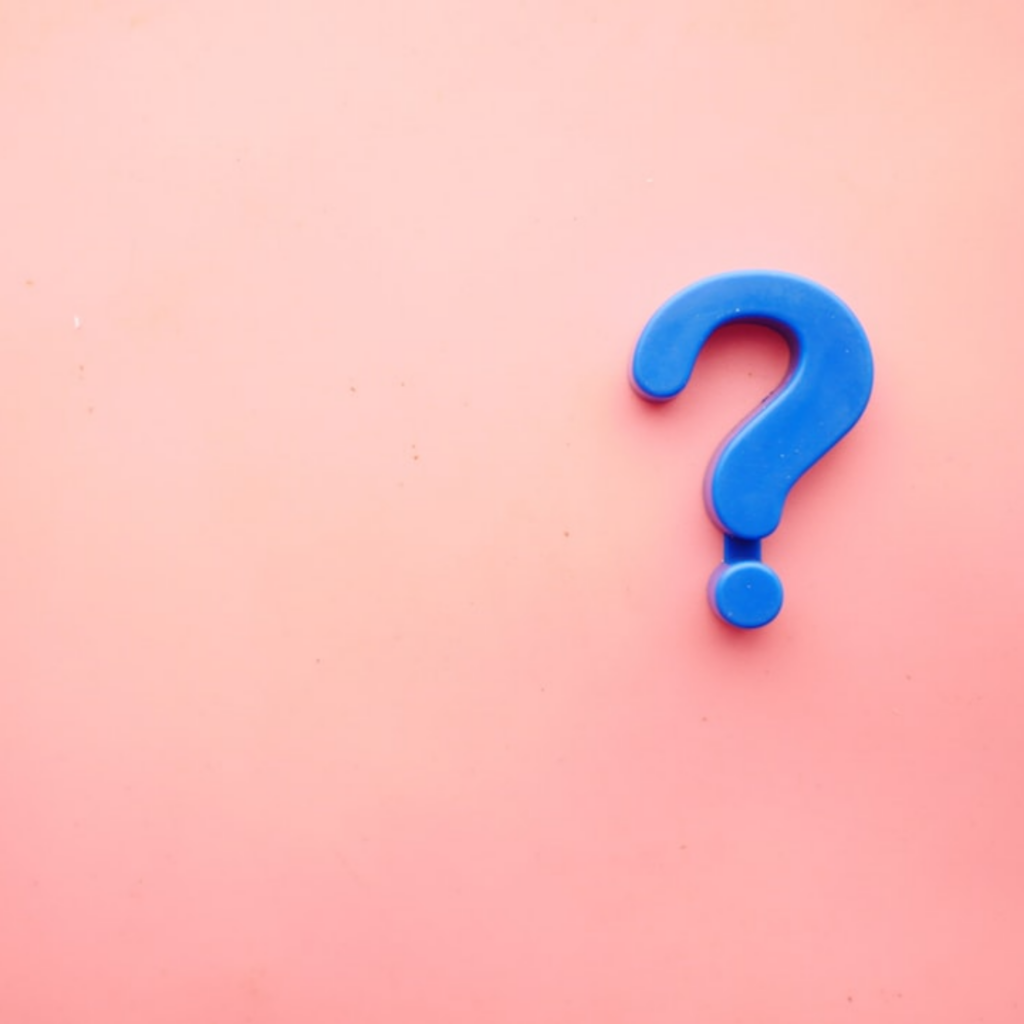
An article published by Oguz A. Acar in Harvard Business Review provides an alternative perspective to what the future of generative AI would look like. He talks at length about the limitations of prompt engineering in AI systems, and how problem formulation would actually be a more holistic approach towards generative AI models.
According to Acar, problem formulation is the ability to identify, analyse, and delineate problems. Both of them differ in their focus, core tasks and underlying abilities. Problem formulation is greatly overlooked and underdeveloped as there is an overbearing importance given to problem solving than formulation itself.
Prompt engineering is highly dependent on an individual’s grasp of a certain AI tool, since Generative AI currently focuses on key words and phrases that the model catches and optimises through its databases. Problem formulation on the other hand prerequisites a sound understanding of the problem itself and its domain and produces a well-formulated problem before focusing on the solution.
So when one has a real, clearly-defined problem, the linguistic specifications of the prompt become secondary in the solution.
Conclusion
Prompt engineering is highly important in generative AI right now, but it is important to understand its limitations in order to understand generative AI better. Moreover, problem formulation as a skillset should also be worked upon, as it may stand to be equally, if not more, important as prompt engineering in the future of generative AI.
It is an exciting time to be in tech right now, and there are no limits to the imagination of how the future of tech would look like!